Prediction of machinery degradation in Cogniplant not so simple
- Alfons Salden
- Mar 31, 2022
- 2 min read
On the one hand, MRNEC develops deep learning models to support the project's use case partners in better understanding and predicting production processes. These models require formulation of a so-called supervised learning problem with respect to historic and actual data streams concerning process values, control actions and set points. Thereto, MRNEC applies machine learning next to multi-dimensional time series data analysis techniques. On the other hand, MRNEC contributes to the development of decision support tools for just-in time rescheduling of the control of production processes of the use case partners in the project. All this with the ultimate goal of improving these production process parametersof the use case partners for certain key performance indicators.
For example, in Acería de Alava (ACVA)) use case the challenge is predicting and optimizing the remaining useful lifetime of grinding machines during steel tube production. This is not to say almost impossible a very difficult problem as complex as controlling the plasma in a nuclear fusion reactor, because their lifetimes depend on a very complex interplay of individual bearing production and operating aspects.
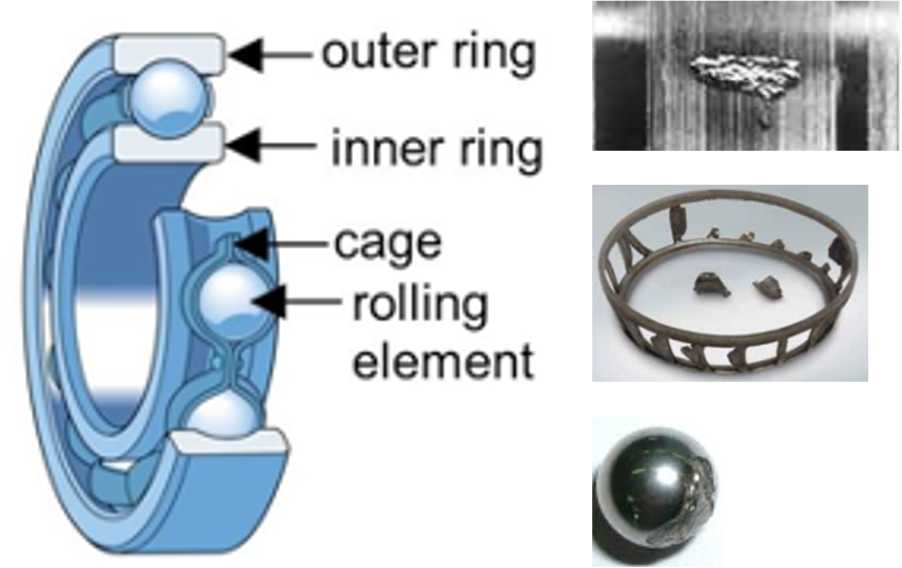
During grinding operations, the required load, running speed, possibly lubrication to minimize friction and temperature cooling need to be adapted to the specific type and quality of casted steel work pieces at hand. In turn, however, these operating conditions related to the manufactured product impact the wear and cracks or fracturing by fatigue and thus the overall performance degradation of the bearings. Misalignment and unbalance in the grinding machines due to structure design or assembly flaws also significantly affect the bearing wear and fracturing. Needless to say, structural quality and defects of the bearing components (outer and inner rings, cage and rolling element) lead in combination with such operating, design and assembly aspects to a possibly rapid decline but often idiosyncratic evolution of its defects and their interaction. Under such circumstances that are determined by shaft speed and load, the time series data of the horizontal acceleration of the same type of bearings display completely different RUL times showcasing perfectly the difficulty in mastering having grip on such degradation processes.
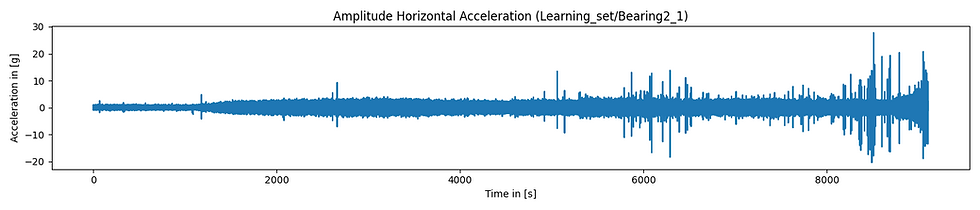

Comments